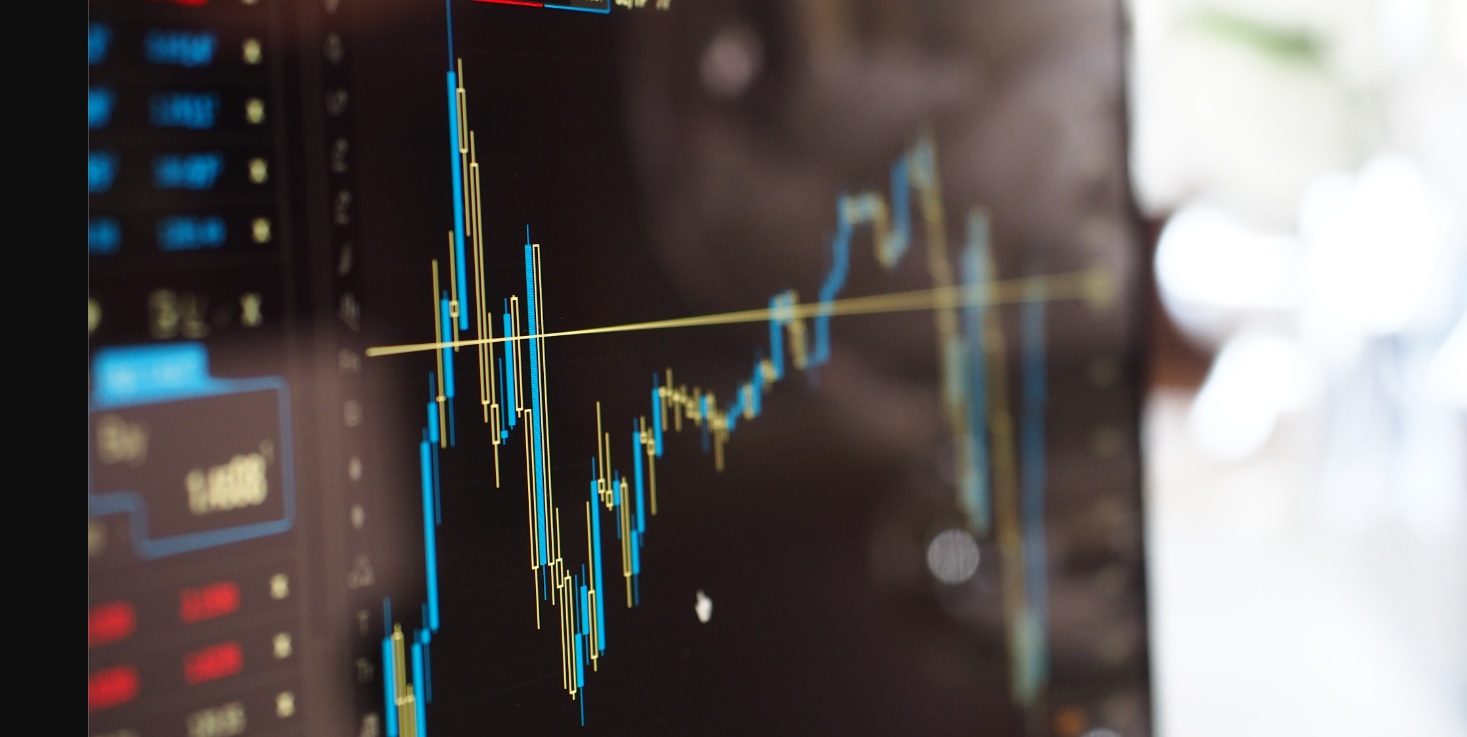
In recent years, artificial intelligence (AI) has garnered significant attention for its potential to revolutionize various industries. One area where AI has been heavily explored is stock trading, with promises of smarter decisions and higher profits. However, the reality seems to paint a different picture: AI is struggling to effectively navigate the complexities of real-world stock trading.
AI algorithms are designed to analyze vast amounts of data, identify patterns, and make informed decisions based on historical trends. This capability has shown promise in backtesting environments and controlled simulations. But when deployed in the dynamic and unpredictable realm of real-world stock markets, AI often falls short.
One of the primary challenges faced by AI in stock trading is the inherent unpredictability of markets. Financial markets are influenced by a multitude of factors, including geopolitical events, economic indicators, and even public sentiment. These variables can swiftly shift market dynamics, rendering AI’s historical analysis insufficient for accurate predictions.
Additionally, the “black box” nature of AI poses challenges for transparency and accountability. Traders and investors find it difficult to fully understand the decision-making process of AI algorithms, leading to concerns about biases and errors that might go unnoticed until it’s too late.
Another crucial factor is the element of human emotion. While AI is designed to be rational and objective, human traders are often influenced by psychological factors that AI cannot fully emulate. Fear, greed, and intuition play a substantial role in decision-making, often leading to outcomes that defy logical analysis. AI’s inability to incorporate these emotional nuances can lead to suboptimal trading decisions.
Lack of adaptability is another concern. AI models trained on historical data might struggle to adapt to new market conditions or structural changes. This limitation was evident during the GameStop stock frenzy, where AI algorithms failed to predict or respond effectively to the surge driven by online communities.
Despite these hurdles, proponents of AI in stock trading remain optimistic. They contend that ongoing improvements in AI and machine learning algorithms may eventually result in more efficient tactics. Additionally, their capacity for real-time AI model adaptation could aid in more intelligently adapting to shifting market conditions.
In conclusion, while AI has shown promise in controlled environments, its application to real-world stock trading has exposed significant challenges. The unpredictable nature of financial markets, the human element, and regulatory considerations all contribute to AI’s struggle to generate consistent success. As technology continues to evolve, it remains to be seen whether AI can overcome these obstacles and truly transform the landscape of stock trading.
Leave a Reply